Make Breakthroughs, to Make Millions, with Two-Level Factorial Experiments
Whether your business relies on a process or system for fabricating solar cells or launching email marketing campaigns, you need to identify the factors that make a difference and optimize them. Do you have a complicated system or process in your business that isn’t delivering the results that it should? Do you need to get a handle on the key factors at the heart of the system or process for your business? Is your systematic approach of conducting A/B split tests simply not revealing the important factors, nor their optimum settings? Are running out of time, money and patience with all the A/B split tests that don’t seem to you anything?
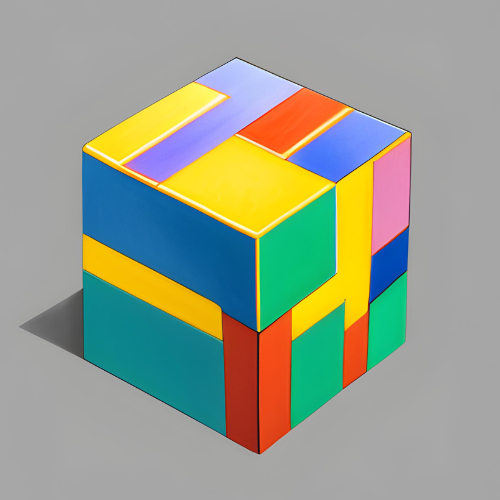
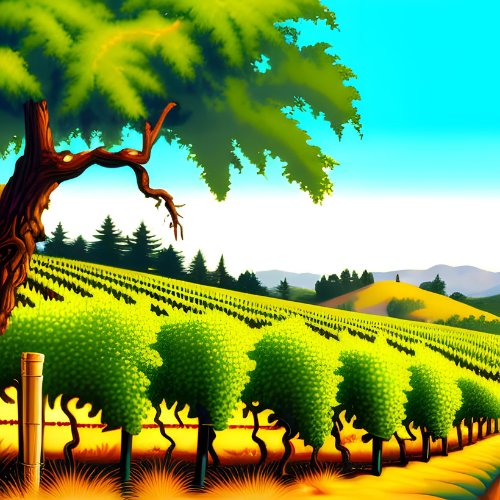
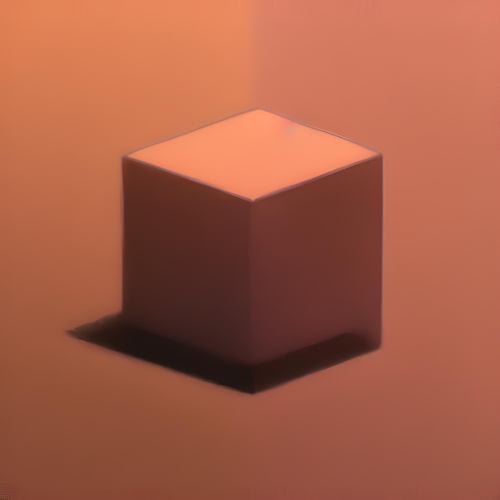
A Far More Efficient Alternative to A/B Split Testing
Imagine running a series of tests to efficiently survey several factors for your system or process. Let’s imagine they reveal the factors that matter far more efficiently than testing one factor at a time by a series A/B split tests. Moreover, the tests could reveal the interactions between factors. With a few additional tests, the tests could also reveal the optimal settings for the critical factors. And you could do all of this without interrupting the normal flow of production or sales because the tests overlay onto your routine process or system of business or production.
Well it turns out that you can systematically alter several factors at a time over a definite set of tests in which you efficiently cover all the possible combinations of changes to those factors. If you devise the changes so that they’re big enough to create a measurable difference, yet not too far off from the original to hopelessly degrade the result, then the overall average of your test results can serve as an internal control while all of the measurements contribute to a realistic estimate of the inherent noise in the experiment, for gauging precision. Moreover, the results will reveal how the process responds to the variation of each factor in combination with the variation of the other factors, in terms of magnitude and statistical confidence. All can be extracted from a minimum number of tests at a minimum cost.
What’s required is a framework or design for the series of tests.
Let’s Begin with the Two Level Factorial Experiment
Fortunately there are scores of proven designs that are routinely used to accomplish what I just described. You can select the best set of experimental designs for your process or product based upon how many factors you need to investigate, whether those factors are quantified or categorical, and whether or not you need a granular or detailed view of how the process responds to changes in those factors. In this article I’m going to focus on the two level factorial experiment design for factors that can be varied between high and low levels, on or off states, and present or absent. The experiment can be performed for several independent factors at a time. In fact the more independent, orthogonal or unaliased the set of factors, the better.
Benefits of Two-Level Factorial Experiment over A B Split Testing
Save time and money
The whole motivation behind DOE in general, and the 2-level factorial experiment in particular, is to gain the greatest amount of useful information from the minimum number of costly tests. In other words, it’s about getting the best return on investment from your expensive process and product experiments. It enables you to take far fewer tests while exploring multiple process factors and how they interrelate. These benefits set DOE above the practice of varying a single factor at a time, or of comparing only two sets of conditions at a time as for A B split testing.
Moreover, a DOE approach using 2-level factorial experiments enables you to quickly isolate the most important factors by providing you with the best signal to noise with the fewest number of samples.
More info from your data, more reliably
In addition to helping you isolate the important factors, a 2-level factorial experiment lets you gauge the relative impact of those factors without having to worry so much about experimental drift, or how unknown or uncontrolled factors drift during the course of the experimental campaign. DOE also enables you to ferret out how pairs of factors may interact.
Practical Utility and Profitable Insight
Using DOE techniques, you can easily extend or augment your two level factorial campaign with tests to include axis points and center points. These will enable you to account for curvature as you draw a map or build a response model that reveals the optimal set of conditions or the most stable settings for factors that matter.
Additional Benefits
Description of Additional Benefits
More Insight into the Two Level Factorial Experiment
Let’s consider what kinds of factors you can explore in a two level factorial experiment.
First of all, you can include all of the factors that you’d normally test in an A B split test where you compare the effect of adding an element versus not adding an element, or compare the difference between two different versions of an element. The key is to define the presence or absence of an element, or version 1 and 2 of that element, as a separate factor.
Beyond that, you can test low and high levels of all continuously or stepwise variable factors that can be measured along a scale, whether a qualitative rating or a quantitative scale.
Essentially, you want to choose two distinct cases or levels for each factor that provide contrast within the limits of practical productivity.
What I aim to do in this article is to win you over to the benefits and advantages of one of the mainstay tests from the world of Design of Experiments – the two level factorial experiment.
Essential Procedure
Define and State the Objectives of Your Testing
In the grand scheme of things, it’s best to begin by stating the objectives of your tests by listing all of the different factors that you can control along with your best attempt or guess at listing them in decreasing order of priority or importance to the process or product. You also want to specify those special factors that you’d prefer to keep a certain level, perhaps minimum or maximum, in order to save money, time or sanity. The third objective you need to state or write down is the desired outcome in terms of the effects that you’ll be measuring. Again, it’s helpful to list the desired outcomes up front in decreasing priority. Finally list all of the factors that need to be kept constant and the corresponding reason for doing so.
Ultimately you will have a prioritized list of all your factors along with each of their two extreme levels or contrasting cases. For example, you could test a call to action button on a web page according to the following factors: size (small, big), color (green, red), visibility (low, high), page position (ATF, BTF). Similarly, you could test the efficiency of the design of a diesel engine in terms a series of factors such as compression (low, high), fuel/air mix (lean, rich), revolutions per minute (minimum, maximum), piston stroke (short, long), piston area (small, large).
Do your best to choose factors that are independent to avoid having to perform an unwieldy number of tests. For example, consider the visibility of a call to action button. Its color, size and position are all independent factors that determine its visibility. But defining visibility as an additional factor would be redundant because visibility is not independent of the other three.
It always pays to apply the Pareto Principle or the 80:20 rule to your list of factors. Try to identify the top most important 3 or 4 factors from your list, or better yet, the top 2 or 3 factors. By the Pareto Principle, half of the effects are accounted for by a minority of factors, approximately the square root of the total number of factors.
List the Control Factors
List all the important factors and identify their low and high settings or limits that are far enough apart to show a difference but not so extreme as to completely shut down sales, kill the tomato crop or make the pizza inedible.
If possible, select the top 3 most important factors likely to make the biggest difference. Set all the other factors to their best known settings.
Figure out how to gauge the response of the experiment – Measure everything you can manage.
Sometimes the response may be clear cut, unambiguous and quantitative. For example, you can measure the total weight of tomatoes produced by a crop. In other cases, you may need to define a rating score or quality score that depends on subjective opinion. For example, you may need to assess the flavor of the tomatoes from your crop.
If you need to rely on subjective qualities, it often helps to tally up the scores for a number of component impressions that comprise the overall quality. For example, the taste score for tomatoes can be tallied up from separate scores for mouth feel (powdery = 1, crisp and clean = 10), tartness (bland = 1, tart = 10), flavor intensity (weak = 1, rich and deep = 10) and freshness (“off” tasting = 1, super fresh = 10). It also helps boost the reliability of your scores to take the average result from several observers, or a team of volunteer tomato tasters.
Choose an Efficient Design for Your Experiment
Once you’ve got the important control factors and the outcome measurements all sorted as I’ve described above, it’s time to choose the best experimental design for gauging the magnitude of the effects of varying the control factors.
Test combinations of high and low levels:
Two Factors:
Low Low
Low High
High Low
High High
(Mid Mid)
Three Factors:
Low Low Low
Low Low High
Low High Low
High Low Low
High High Low
High Low High
Low High High
High High High
(Mid Mid Mid)
List the Response Factors
To get the most out of your experiment, be sure to measure everything you can practically manage to record that may make a difference to your ROI. For the tomato crop example, record your overall costs for garden area, water, fertilizer, storage and labor, et cetera. Also gauge the shelf life of the fruit, losses to pests and disease, and all the other measures that are relevant and significant to the outcome of your tests.
It’s sometimes better to conduct the tests in random order but not essential unless there are significant, unknown, uncontrolled factors (e.g. drift)
You can then use widely available software to easily perform statistical analysis and generate a report.
Run a series of factorial experiments
Plan on an iterative series of experiments. Begin by surveying control factors over the widest range. For successive iterations, eliminate the control factors whose effects are insignificant while narrowing the range of the experiment to a smaller region of interest.
Use Software the Extract Knowledge from the Experimental Data
Apply a variety of statistical tools to eke out clues from your experimental data. Use Q-Q plots to identify the most important effects. Use a variety of tests to detect outliers in your data, then disclude those outliers from further analysis. If required, apply mathematical transformations to your data to help bring out signals from the noise. Use analysis of variance to evaluate the significance of effects for each factor, interactions between factors and higher order terms. Define a model based on a polynomial with terms for the significant factors, their interactions and higher order, quadratic and cubic terms. Fit a polynomial function, or other appropriate mathematical function, to the experimental data by least squares regression. Finally, evaluate the statistical significance of the model. Use the results to report the optimal range of control factors.
Examples:
When growing tomatoes, begin by finding the best combination of sunlight, water volume, and fertilizer for your crop by conducting a 2 level, 3 factor experiment. Include midpoint tests. Then move on to find the optimum levels for the following: min/max soil pH, min/max N-P-K (each), min/max watering frequency, min/max shade protection, min/max pruning, min/max soil volume/area per plant, et cetera
Find the optimum combination for your advertising sales copy – short through long form/sales video length, minimum through maximum CTAs, min/max number of benefits, min/max number of pain points, low/high grade level for readability.
Discover a killer pizza recipe by finding the optimum combinations of the following: water to flour ratio, yeast to flour ratio, oil to flour ratio, short/long kneading time, short/long proofing time, amount of tomato sauce, tartness and sweetness of the tomato sauce, amount of seasoning, low and high amounts of specific herbs and spices, amount of cheese, low and high amounts of specific cheeses, low/high bake temperature, short/long bake time.
Software
There are a couple of dozen software packages that can really help with conducting a Design of Experiments test.
Several of the industry leading software options are pretty pricey at more than $1k. Fortunately, these offer a free trial period of a month or two. Only a few really focus on Design of Experiments while many provide vast and comprehensive platforms for statistical analysis and analytics in general. So my warning to you is that when you ultimately buy a commercial software option you may be purchasing a lot of statistical analytical power that you end up never using.
That’s why I recommend looking at free software, free trials and budget friendly add on software options first, or at least the free trial periods, so that you can assess how useful the DOE tools are to your business and whether you only need a stripped down, basic set of DOE tools or the whole shebang, the whole statistical power house.
Let’s start with the freeware open source options that give you the basic ability to analyze the results from a simple two level factorial experiment.
I’m afraid I haven’t yet evaluated any just yet but I have a short list to work through:
Blue Sky (used in conjunction with R)
GNU PSPP
Jamovi
JASP
Python
SOFA (statistics Open for All)
R
A couple of options provide templates or addon code with DOE functionality to MS Excel: Excel Addons – QI Macros $350; Sigma XL $300
There’s even a professional engineering software package whose functionality is dedicated to DOE: Design Expert
Finally, there are statistical powerhouse software options that come with everything statistical except the proverbial kitchen sink: JMP, Unitab, Minitab
Barrier to Adopting
As I have already hinted, I believe that the barrier to adopting some of the advantageous and effective experimental designs over the simple A B split test is that it creates a hurdle of communication that needs to be overcome through persuasion and explanation. That barrier seems to be enough to banish it to the rare organizations where a critical mass of employees are already familiar with the competitive advantage offered by more efficient and effective experimental designs. Otherwise, why would you go out on a limb unless you were absolutely desperate to turn around an inefficient process or sales funnel and make it profitable?
This might actually be closer to the truth than I suspect. After all, the whole discipline of Design of Experiments really began to explode on the scene during the tense and desperate years of lean economy of world war II when every action on the home front needed to make the absolute best use of resources.
That’s the ethos or credo or purpose behind Design of Experiments, to extract the most useful insight and information from the smallest investment into tests and process experiments.
But now we are coming to the end of several decades of wealth and abundance when it has become commonplace to throw an incredibly large budget at optimizing a sales funnel or refining a product or process to get the absolute highest quality or perceived customer value.
If these are the reasons then I guess that the adoption of Design of Experiments must be cyclical.
So how do I make the most persuasive argument in favor of adoption?
One thing I can do is perform an experiment to see what benefits and solutions can be sold to my prospects to outweigh the inconvenience they might face in educating their peers.
The other thing that I can do is to actually go out and ask people if they know about Design of Experiments, if they’ve had positive results with it, and whether they are satisfied with the limitations and ROI for their current A B testing. I can put together a little online survey with these and other questions to really get to the bottom of the resistance towards Design of Experiments.
Why Resort to “Design of Experiments” ?
The devil is in the details and that’s where testing and experimenting comes in.
Observance of the Experimental Control
We’ve all learned that you need to control the conditions of an experiment as far as possible, vary only one factor at a time, then compare the result with a fixed “experimental control.” Indeed, the leading method for testing factors is to perform an A B split test, where you change a single factor and compare the result with the experimental control. In other words, the leading method is to vary one factor at a time. The “A” run of the experiment is usually the fixed experimental control, while the “B” run involves changing a single factor to a lower or higher level, an on or off state, or a present versus absent version, or different state such as different color, in order to gauge the effect of that factor. Then you must repeat the A B split test to gauge statistical variation of the result relative to the average effect. The important principle is to change only one factor, in one respect, and compare the result to the experimental control, until you obtain a measure of the average effect and the inherent level of random variation or noise. Otherwise, how can you tell which factor is responsible for the new result, or whether or not the new result is due to noise?
The problem with taking this approach is that there is scarcely the time and money to evaluate all the factors in play in terms of effects and noise.
Experimenters Still Shy Away from DOE
After seeing solid proof first hand of how design of experiments can take a process or product out of the weeds and deliver something market worthy, I am frustrated to see how marketers and entrepreneurs all seem to prefer A B testing, and one factor at a time experiments, to the exclusion of any other type of test, no matter how much more efficient and cost effective the other types of tests and experiments may be.
I don’t precisely know what the barrier is towards making the leap from A B split testing to many of the standard Design of Experiments (DOE) tests that are available. I suspect that perhaps people simply aren’t aware of the DOE types of tests, or that they perceive DOE tests to be overly complex, or perhaps they’re shying away from the need for for statistical analysis software to interpret the DOE results, or perhaps it’s the fear that it will be too hard to communicate the results effectively to decision makers.